MCP and the AI Governance Gap: A Wake-Up Call for Enterprise
Anthropic's Model Context Protocol (MCP) is gaining significant attention in the AI community. But is it pushing some important governance issues into the background?
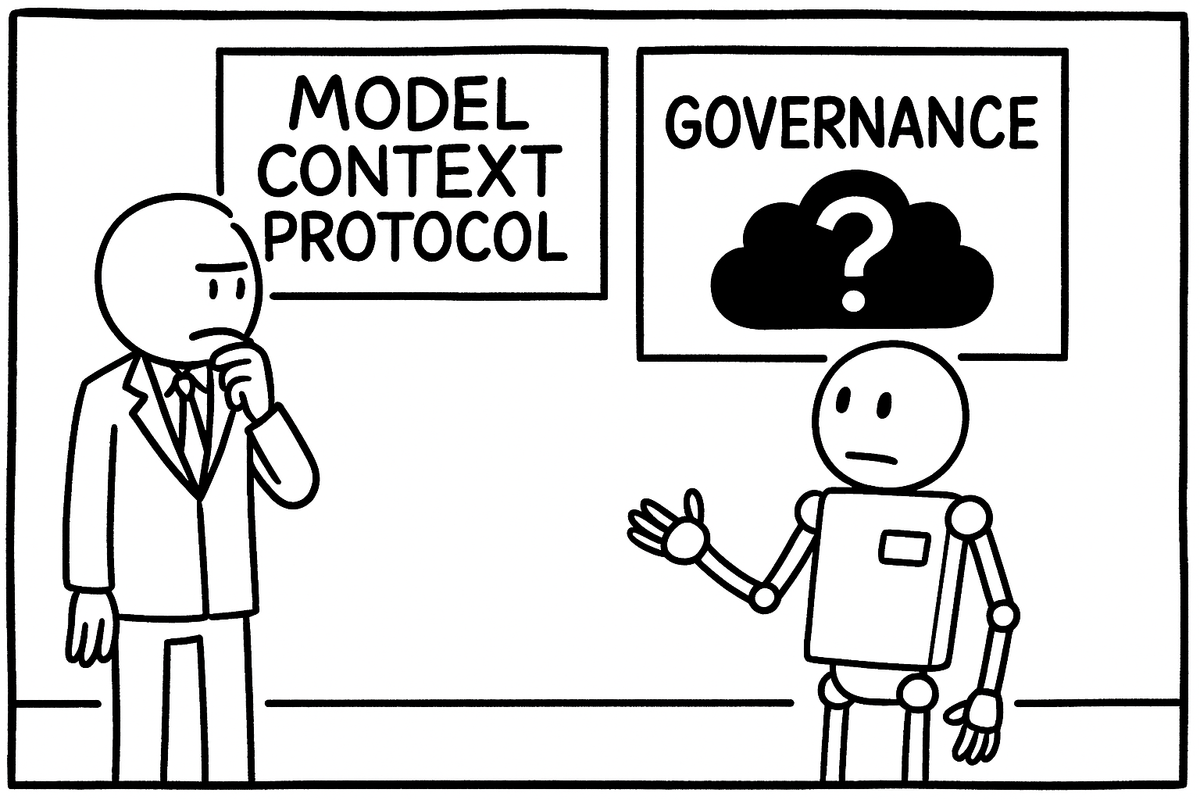
I spent some time on the weekend watching a workshop presented by Mahesh Murag of Anthropic. It provided valuable insights into MCP's capabilities and potential impact. For those involved in AI decision-making within organizations, especially concerning AI agents, this development is worth keeping an eye on.
MCP is an emerging standard designed to facilitate seamless integration between large language model (LLM) applications and external data sources and tools. Standardization in software development is beneficial as it promotes consistency, interoperability, and efficiency. The adoption of MCP is poised to accelerate the development and deployment of AI agents across various domains.
One comment in Mahesh's presentation really stood out, however: "People are still working out how to do data governance on this." This observation highlights a critical area of concern. While MCP provides a robust framework for integrating LLMs with diverse data sources, it does not inherently address fundamental governance aspects - even something as fundamental as user access control.
It's important to note that MCP's architecture does not preclude the implementation of appropriate mechanisms like Role-Based Access Control (RBAC). Components such as the Sessions object and the Sampling back channel offer avenues to gather the information necessary for enforcing such controls. Moreover, many context sources interfacing with MCP servers will inherently possess data provenance features and built-in access controls that can be seconded for the purpose.
Nonetheless, the integration of LLMs and AI agents will obscure data provenance processes, rendering them less transparent. This diagram from the Mahesh's presentation illustrates the problem. All three MCP servers can use LLMs and can be autonomous.
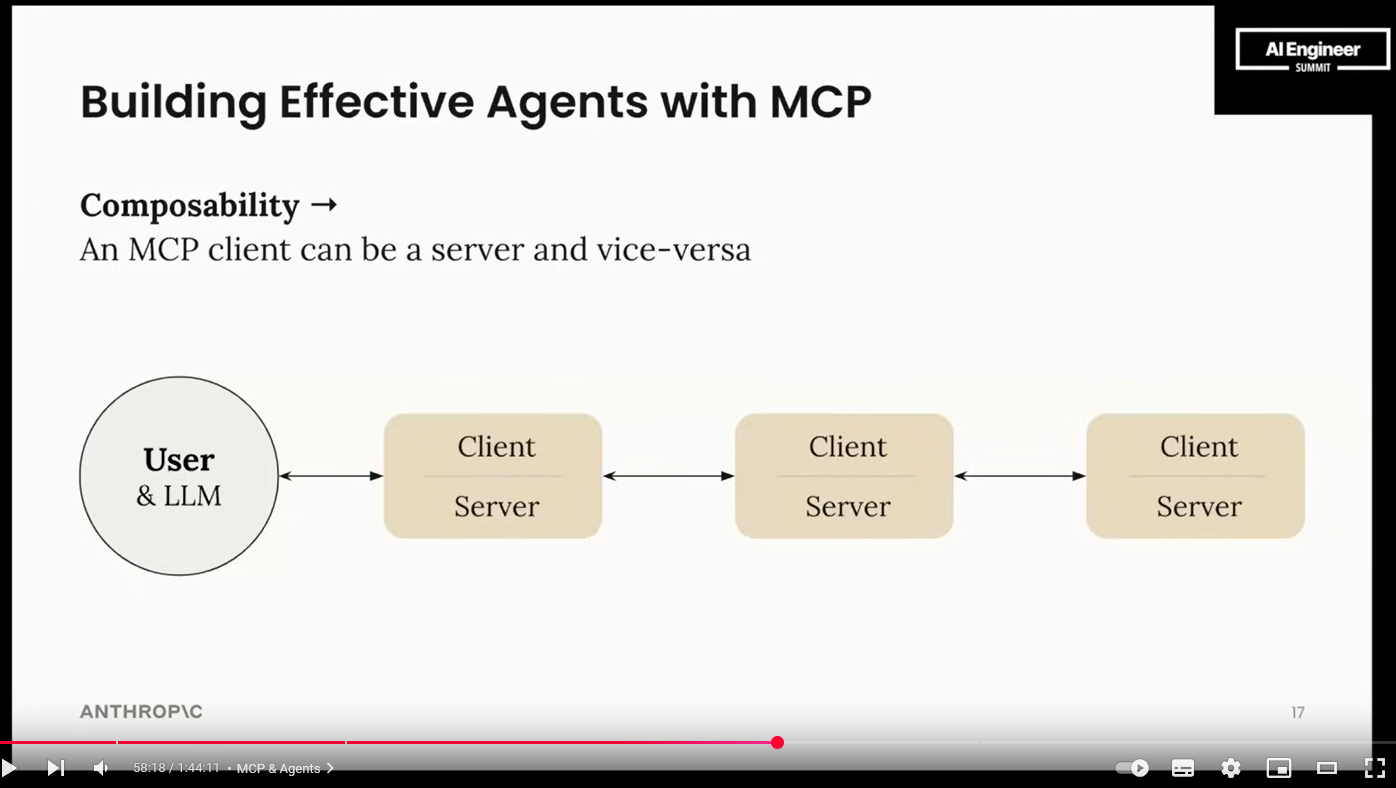
This opacity poses challenges for enterprises aiming to maintain stringent data governance standards. As MCP continues to evolve and gain traction, it is important that organizations proactively address these governance gaps.
If you're looking for a framework to help you ask questions about AI governance in your organization, this checklist is a good place to start.